The Cost of AI Development: What You Need to Know Before Building Your AI Solution
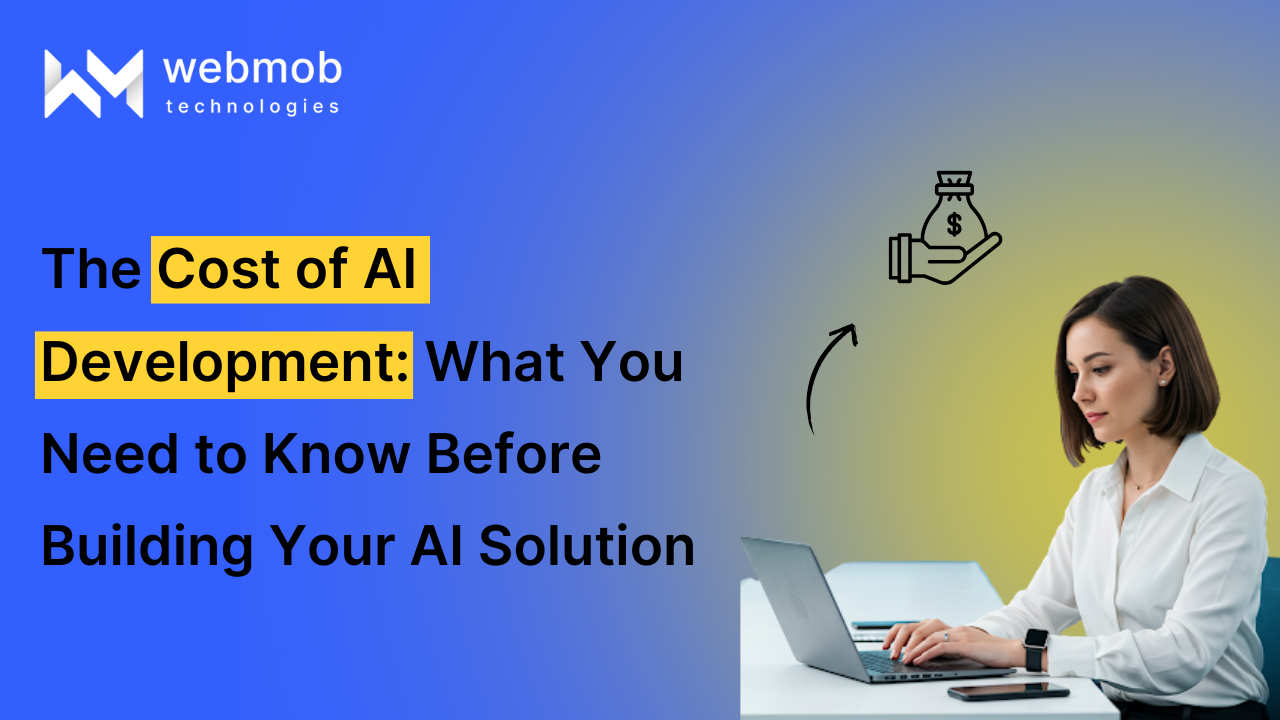
There’s really something captivating about Artificial Intelligence, isn’t there? I mean, the allure is just undeniable right now. Businesses, understandably, are really eager to harness its power – they’re looking to improve efficiency, maybe gain some new insights, and honestly, just innovate. But then the question quickly comes up, the practical one: how much does all of this actually cost? Getting a handle on AI development expenses is honestly pretty crucial for success.
Look, there’s no simple, single answer to that “AI pricing” question. The costs can vary wildly, and it really just depends on the project’s complexity and its specific requirements. It’s kind of like asking “how much does a house cost?” – I mean, it totally depends on the size, the location, the materials you want to use, right? If you’re keen to delve a little deeper into the core concepts of AI generally, exploring resources like Google AI can be a good starting point, I think.
What we’ll try to do here is give you a relatively comprehensive overview. We’ll attempt to unravel some of the complexities around AI development costs. You’ll get a sense of the factors that really influence your AI project budget. The idea is that this will hopefully enable you to plan your project a bit more effectively.
And you know, navigating these complexities is something we at WebMob Technologies have quite a bit of experience with. We’ve helped businesses understand and manage their AI development investments pretty successfully, I think.
Why Understanding AI Development Expenses is Critical
Getting a clear handle on AI development costs isn’t just about the initial price tag, you know? It’s really vital for a few key reasons. We’re talking about things like, crucially, avoiding those dreaded budget overruns and making sure you actually see a strong return on your investment, or ROI.
Honestly, budget overruns can totally derail a project. You know how it is, unforeseen expenses seem to pop up and just drain the funds before you know it. But really getting a handle on what those potential costs could be? That allows for, I guess, much better contingency planning.
And yeah, making sure you get a clear ROI is just as important. You’ve got to be able to justify putting money into AI, right? That means really digging into what the potential benefits are and stacking them up against what you expect to pay.
It just makes planning and figuring out where to put your resources so much easier. I mean, when you actually know where the money is going, you can put those resources where they’ll do the most good. Ultimately, that just makes for, well, better project management overall.
Deconstructing the ‘AI Development Costs’: Key Factors at Play
So, what actually drives these costs? Well, quite a few key factors really influence things. These factors range from the project’s complexity all the way to the data requirements. And honestly, if you don’t pay attention to them, your budget estimates can end up being pretty off.
Let’s try and break them down a bit:
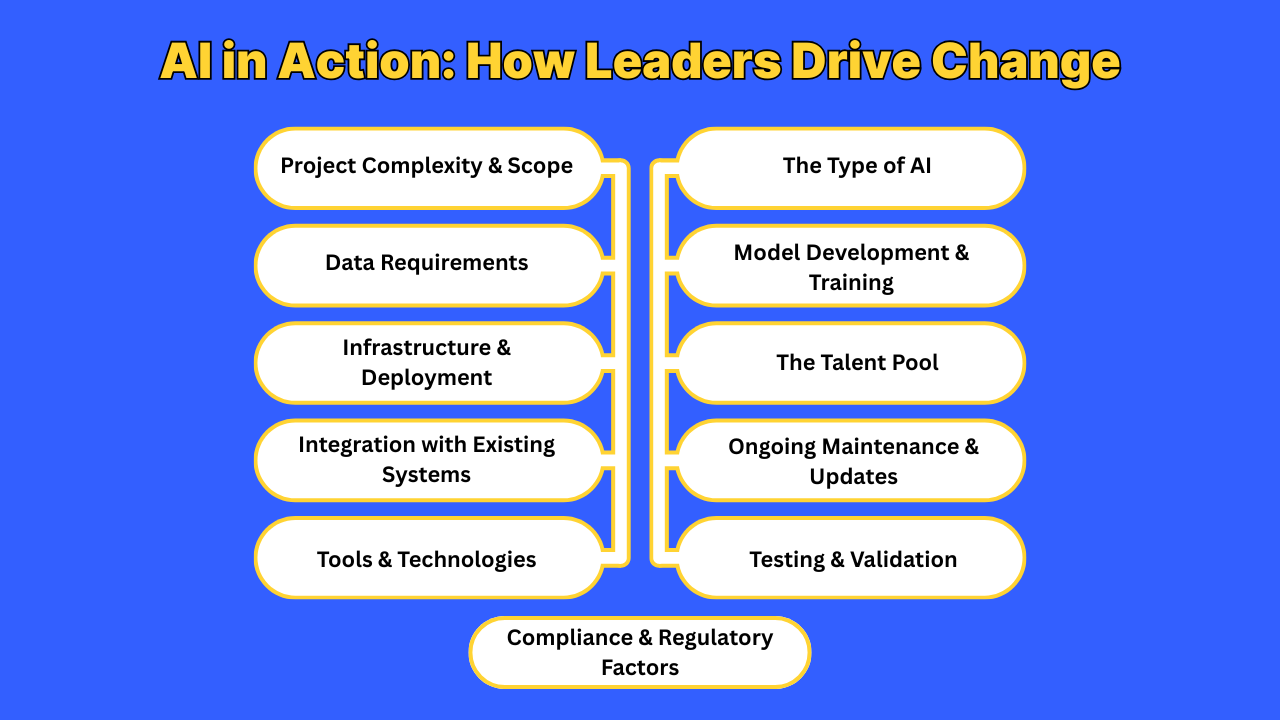
1. Project Complexity & Scope:
Okay, first off, you’ve really got to define the problem. I mean, is it something pretty simple, like just automating a basic task, or are we talking about complex prediction here? A simpler task is naturally going to need fewer resources. But yeah, complex projects? They just demand way more resources, and, you know, cost more because of that.
The number of features you want built in definitely affects the cost too. More features generally mean more development time, that makes sense. And the desired accuracy level plays a role too – higher accuracy often requires more sophisticated models, which usually leads to more cost.
Things like real-time processing needs are different from just batch processing. Real-time applications almost always need more powerful infrastructure behind them.
2. The Type of AI:
Different types of AI tend to have varying costs associated with them.
- Machine Learning (ML): Supervised, unsupervised, and reinforcement learning – each of these has distinct resource needs.
- Deep Learning: Neural networks, CNNs, RNNs, and Transformers are typically computationally intensive, so they can get expensive.
- Natural Language Processing (NLP), Computer Vision, Generative AI: These often require pretty specialized expertise and specific tools.
Choosing off-the-shelf models can sometimes reduce costs, that’s true. But, custom solutions usually provide greater flexibility, though they do come with increased ‘AI model cost’, naturally.
3. Data Requirements:
Data, you hear it all the time, it’s the fuel for AI. And honestly, the quality and the sheer amount of data you have? That directly impacts the costs.
- Data Collection: How easy is it to get the data? The availability, the sources you need, whether you need APIs or scraping – all of that influences the cost.
- Data Cleaning & Preprocessing: Handling missing values and inconsistencies takes time and requires expertise, so there’s a cost there.
- Data Labeling & Annotation: This can be done manually or automatically, and it’s often a pretty significant ‘AI development expense’.
- Data Storage: Where you store your data – cloud vs. on-premise – definitely has cost implications.
4. Model Development & Training:
This is obviously a pretty crucial step in AI development. The cost here is influenced by several factors.
- Algorithm Selection and Experimentation: This requires skilled data scientists, and it can be time-consuming work.
- Training Data Volume & Quality: Impacts the ‘AI model cost’ directly, as we mentioned.
- Computational Resources: Things like GPUs, TPUs, and cloud compute costs really drive the overall ‘AI pricing’ during training.
- Hyperparameter tuning and model optimization: This is an iterative process, often demanding a significant amount of time and compute power.
5. Infrastructure & Deployment:
The infrastructure you choose for your AI solution makes a big difference to the cost.
- Cloud vs. On-Premise: Each has different upfront capital expenses and ongoing operational expenses.
- Cloud Providers: AWS, Azure, and GCP all offer various AI/ML services, and they have different pricing structures you need to look into.
- Environments: Development, staging, and production environments each need separate resources, adding to the cost.
- Containerization: Using things like Docker and Kubernetes adds a layer of complexity initially, but it can really help with scalability later on.
6. The Talent Pool:
Having the right talent is absolutely essential for a successful AI project, I think.
- Data Scientists: Expertise in specific areas like NLP or Computer Vision tends to influence salary expectations.
- ML Engineers: Building the necessary pipelines, handling deployment, and setting up MLOps require specialized skills.
- Data Engineers: Creating and managing the data pipelines is pretty critical work.
- Domain Experts: Often essential for truly understanding the problem you’re trying to solve and validating the results your AI produces.
- Project Managers & QA Engineers: You definitely need these roles for successful project delivery, ensuring things stay on track and work correctly.
The team size and where people are located significantly affect ‘AI development expenses’. Onshore, offshore, and nearshore options all have quite varying cost structures. You know, this is actually an area where we think we help quite a bit at WebMob. Our team structure is pretty diverse, and we really try to optimize for talent costs, getting you the right expertise without necessarily breaking the bank.
7. Integration with Existing Systems:
Integrating your new AI with existing systems… yeah, that can be pretty complex sometimes.
- APIs and legacy systems: The complexity of the systems you need to integrate with definitely affects the effort required and, therefore, the cost.
- Data flow: Ensuring seamless data flow between systems is absolutely crucial for your model to actually be used successfully in the real world.
8. Ongoing Maintenance & Updates:
Look, AI models aren’t a ‘set it and forget it’ thing. They really need constant care after deployment.
- Model monitoring, retraining, and performance decay: Requires continuous effort to make sure the model is still performing well.
- Software updates and security patching: Essential stuff for keeping things secure and stable.
- Infrastructure scaling and management: This is just part of the long-term ‘AI pricing’ picture.
9. Tools & Technologies:
Choosing the right tools for the job is pretty important.
- Software licenses: Platforms, libraries, and MLOps tools often come with licensing fees you need to factor in.
- Open-source vs. proprietary: Each has its own cost implications, obviously.
10. Testing & Validation:
Thorough testing is crucial before you deploy anything important.
- Testing frameworks: Establishing robust frameworks for testing is vital, though it takes effort upfront.
- User Acceptance Testing (UAT): Ensures that the solution actually meets the requirements of the people who will be using it.
11. Compliance & Regulatory Factors:
These factors can definitely add to the cost, and they’re important to consider.
- Industry-specific regulations: Industries like healthcare and finance have pretty strict requirements you have to meet.
- Data privacy: Things like GDPR and CCPA compliance are absolutely essential these days. And you have to think about ethical AI considerations too, which can sometimes impact development choices and costs.
Typical AI Project Budget Breakdown: Where Does the Money Go?
It’s pretty important, I think, to understand how your budget is typically broken down. Where does the money actually tend to go in an AI project? Here’s a typical breakdown, just to give you an idea:
- Personnel Costs: This tends to be the biggest chunk, often the largest component by far. It includes salaries for those data scientists, engineers, and project managers we talked about.
- Infrastructure/Compute Costs: Can be pretty substantial, especially for deep learning projects that need a lot of processing power.
- Data-Related Costs: Honestly, these are often underestimated. This includes all that effort for data collection, cleaning, and labeling.
- Software/Tooling Costs: Covers those licenses and platform fees we mentioned.
- Operational & Maintenance Costs: These encompass the long-term expenses, the stuff that goes beyond just the initial development phase.
Here is a table representing a simplified breakdown, just for a quick visual:
Cost Category | Percentage of Budget |
---|---|
Personnel | 40-60% |
Infrastructure/Compute | 15-25% |
Data-Related | 10-20% |
Software/Tooling | 5-10% |
Operational & Maintenance | 10-15% |
AI Development Cost Models: How Development Partners Structure Pricing
Okay, so how do development partners actually structure pricing? There are different models out there, and understanding them is pretty key when you’re trying to select the right partner for you.
- Fixed Price: Good if you know exactly what you want from the start, when the scope is really well-defined.
- Time & Material: Offers more flexibility if your requirements might evolve as you go along.
- Dedicated Team: This model is often ideal for longer-term projects that really need integrated resources working closely together.
- Hybrid Approaches: Some partners might even combine elements of different models, which can sometimes work well.
At WebMob Technologies, we really try to work with our clients to pick the pricing model that makes the most sense for them, the one that offers the most suitable and transparent ‘AI pricing’. We aim for clarity and making sure everyone’s on the same page.
Estimating Your ‘AI Development Expenses’: A Practical Framework
So, how do you actually go about estimating these costs accurately? It really helps to have some kind of structured approach.
Phase 1: Discovery & Scoping
- You need to clearly define the problem you’re trying to solve, your objectives, and what you actually want the outcome to be.
- Assess how ready your data is – this is a big one.
- Do a feasibility study to see if your idea is actually viable.
You know, this first phase is so critical. At WebMob Technologies, we often start with what we call Discovery Workshops. It really helps refine the scope and gives you a much clearer initial estimate right from the get-go. Gives you that early clarity on what you need and, importantly, what it might cost.
Phase 2: Detailed Planning
- This is where you select the specific AI approaches and models you think will work best.
- Estimate the effort needed for data collection and labeling.
- Plan out your resources – the talent you’ll need, the compute power.
- Conduct a risk assessment – what could go wrong?
Phase 3: Getting Quotes and Comparing
- Once you have a plan, you can start getting quotes. Make sure you really understand what’s included in each proposal you receive.
- It’s always a good idea to ask potential partners about their pricing assumptions – what are they basing that number on?
Beyond the Initial ‘AI Model Cost’: Long-Term Ownership Expenses
Okay, but it’s not just about the initial build costs, is it? You really can’t forget about the longer-term ownership expenses once your AI is up and running.
- Inference Costs: This is the cost every time the model makes a prediction or processes a transaction. That can add up depending on usage.
- Model Monitoring & Maintenance: Making sure your model is still performing as it should, detecting things like ‘drift’ where its performance degrades – this needs continuous effort.
- Retraining Costs: You’ll likely need to retrain your models with new data periodically to keep them relevant, and that costs money too.
- Infrastructure Scaling: If your user load or data volume increases, you’ll need to scale up your infrastructure, which impacts costs.
- Ongoing security and compliance updates: These are just things that always need to happen, adding to the long-term cost.
Hidden Costs and Common Pitfalls in AI Development
And yeah, watch out for these potential pitfalls. There are definitely some hidden costs that can sneak up on you if you’re not careful.
- Underestimating data preparation costs – seriously, this is a big one for many projects.
- Scope creep without a proper change management process in place.
- Failing to plan adequately for integrating with existing systems.
- Neglecting MLOps and the ongoing maintenance needs after deployment.
- Choosing the wrong technology stack from the start, which can lead to issues later.
- Poor communication within the team or with your development partner – surprisingly costly in delays and rework.
Optimizing Your ‘AI Project Budget’ Without Compromising Quality
Okay, but what if you need to keep costs down? Here are some strategies that can help optimize your ‘AI project budget’, hopefully without sacrificing quality.
- Start small, maybe with an MVP (Minimum Viable Product) to test the waters.
- Leverage transfer learning or pre-trained models where possible – someone else did some of the heavy lifting already.
- Use cloud resources strategically – don’t overprovision, and shut things down when you’re not using them.
- Prioritize features based on their actual business impact.
- Invest in MLOps infrastructure from the start, it saves pain (and money) later.
- Partner with an experienced team that knows how to build efficiently.
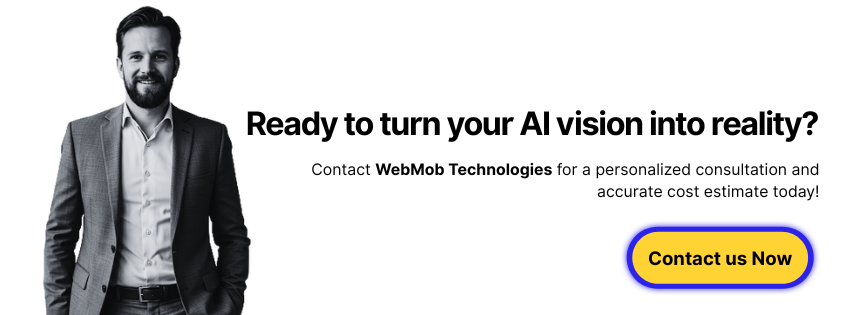
Why Partnering with WebMob Technologies for Your AI Development Makes Financial Sense
So, why consider partnering with WebMob Technologies? We think working with us can actually make a lot of financial sense for your AI project.
- Expertise Reduces Risk & Rework: Our experience means we can help you avoid common pitfalls, minimizing costly errors and rework down the line.
- Accurate Estimation & Transparent ‘AI Pricing’: That Discovery Phase we talked about? It really helps provide much more predictable ‘AI pricing’ and we aim for transparency throughout.
- Efficient Processes & Technology Choices: We’ve refined our processes and know which technology choices tend to be more efficient, helping optimize your ‘AI project budget’.
- Focus on ROI: We’re always focused on delivering measurable business value, making sure your investment actually pays off.
- Seamless Integration & Ongoing Support: We plan for integration early and understand the importance of long-term maintenance and support.
Just as an example, we had a client where we actually helped reduce their AI development costs by, say, 30%. We did that by smartly using pre-trained models and really optimizing their cloud infrastructure based on their specific needs. It’s something we really focus on – delivering cost-effective solutions that work.
FAQs About AI Development Costs
Let’s hit a few common questions people ask…
- What’s the average cost of an AI project? Yeah, that really varies so much depending on how complex the project is. There’s no single number I can give you, unfortunately.
- Does using open-source AI tools significantly reduce costs? It can reduce licensing costs, absolutely. But you still need the expertise to use and manage them effectively, which has its own cost.
- How much does data labeling typically add to the cost? It can be a pretty significant portion, depending heavily on the volume of data you have and how complex the labeling task is.
- Are ongoing maintenance costs predictable? With proper planning and MLOps in place from the start, they can be reasonably estimated, yes. It’s harder if you don’t plan for it.
- How can I get an accurate estimate for my specific AI idea? The best way, honestly, is to engage with an experienced AI development company. They can do a detailed assessment based on your specific requirements.
Conclusion: Navigating AI Development Costs with Confidence
Alright, wrapping up here, AI development costs are definitely complex, there’s no getting around that. But honestly, getting informed and planning properly is just key. You really need to consider all the factors involved – thinking about the initial ‘AI pricing’, all the ‘AI development expenses’ involved, the specific ‘AI model cost’, and looking at the total ‘AI project budget’ over its lifetime. And, I honestly think, having the right partner makes a huge difference too.
So, if you’re ready to talk about your AI vision and maybe get a clearer picture of the investment involved?
Contact WebMob Technologies today for a personalized consultation and detailed cost estimate.