How Predictive Analytics is Transforming the Retail Sector
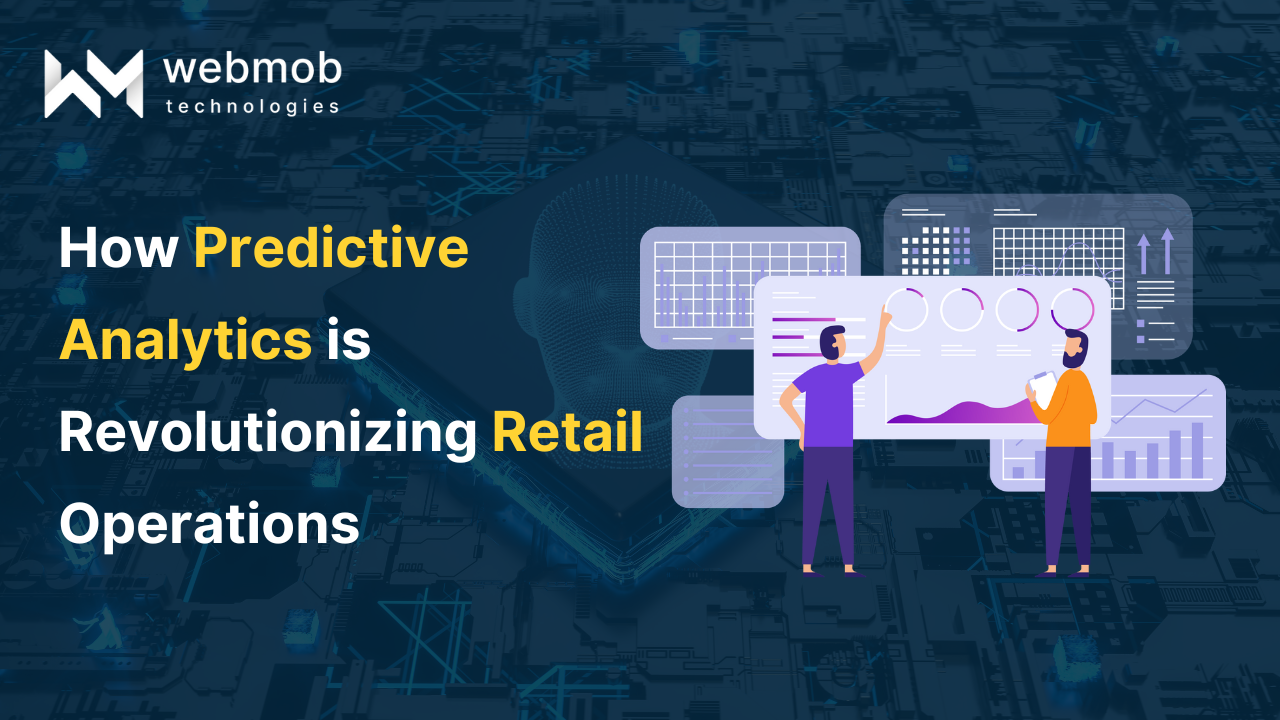
Retailers are constantly striving to deliver the right products at the right time while managing stock efficiently. However, with changing consumer behavior, market shifts, and supply chain issues, this can be a tough challenge. That’s where predictive analytics for retail comes in.
By leveraging data, machine learning, and artificial intelligence (AI), retailers can now anticipate demand with remarkable accuracy, optimize inventory management, and enhance sales forecasting. According to Netguru, 80% of retailers that have adopted predictive analytics have experienced a significant improvement in forecasting accuracy, which allows them to adjust quickly to market fluctuations. A study by McKinsey found that retailers using predictive analytics have reduced supply chain costs by up to 25%. Additionally, the global predictive analytics market is expected to grow at a CAGR of 24% from 2023 to 2030, underscoring the growing importance of predictive tools in modern retail strategies. Retailers who utilize predictive analytics also see a 10-15% increase in inventory turns, helping them optimize stock levels, reduce waste, and maximize profitability.
For more insights into the impact of predictive analytics on retail, check out this detailed article on Netguru’s blog here.
Let’s explore how predictive analytics is transforming the retail sector and how businesses can capitalize on this innovation to stay ahead of the competition.
What is Predictive Analytics in Retail?
Predictive Analysis is the methodology of forecasting future events using historical data through machine learning and AI models. In retail, this simply means predicting customer behavior, sales, and other outside factors, like the weather and trends, influencing decisions at smarter stocks, prices, and marketing.
Retailers can analyze a lot of data to predict items selling well, peak demand periods, and possible quantities needed to keep in stock. This ensures that retailers avoid overstocking, waste, and stock-outs while meeting customer demand.
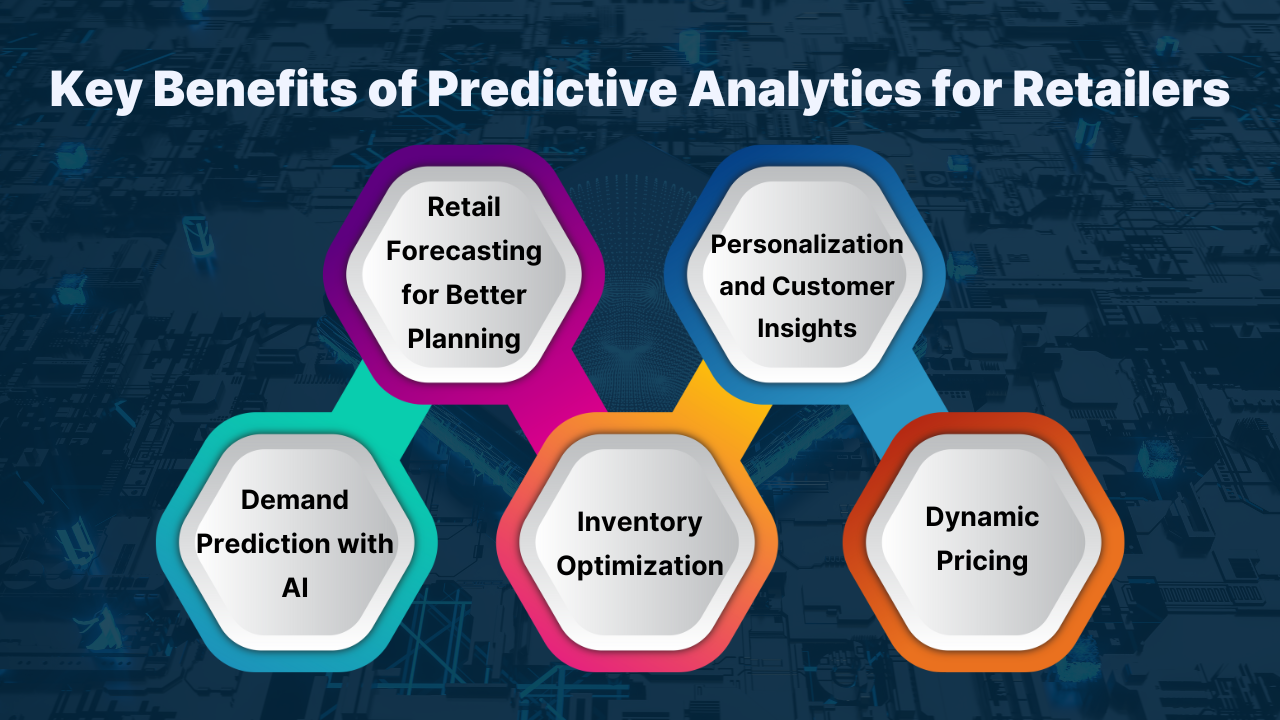
Key Benefits of Predictive Analytics for Retailers
1. Demand Prediction with AI
With predictive analytics, retailers can forecast customer demands with higher predictability. For example, the sales forecast during peak seasons or special local events like black Friday can be generated by the retail organization through AI. They can therefore make arrangements to adjust inventory and provide the right promotions so that they do not run out of popular products or be stuck with unwanted ones.
According to a McKinsey report, demand forecasting through predictive analytics can improve the inventory turnover of a retailer by 10-15%. The ability to develop a clear picture of their products is a key asset as this information enables them to stock the right products and minimize wastage.
2. Retail Forecasting for Better Planning
This is retail forecasting enabling a business to project sales in the future – in general, by using predictive analytics, the retailer can now forecast sales across space, season, and customer segments. Businesses develop a clear vision of their product demand pattern and timing.
This has been stated since accurate retail forecasting has stock optimization, and stockout prevention, and consequently improves the shopping experience of customers. It’s a Gartner estimate that companies would have up to 15% more sales and 20% better inventory management with advanced forecasting technology.
3. Inventory Optimization
Effective inventory management has been one of the biggest retail challenges. Predictive analytics capabilities call forth the anticipation of demand for specific products at specific times. The most effective mode of demand generation, from the perspective of continuous operations, is to ensure stock levels are in real-time. For instance, AI agents can automatically adjust inventory orders based on sales data to avert both stocking and out-of-stock.
Retail businesses could know when products would sell out and automatically trigger reorder alerts. By having the right products at the right time, retailers can avoid lost sales and improve customer satisfaction.
4. Personalization and Customer Insights
Predictive analytics is a tool that helps retailers understand customer preferences and behaviors. It analyzes data from past purchases, browsing habits, and demographics to be able to provide personalized recommendations, promotions, and product suggestions for a customer.
For example, if a customer often buys a specific kind of product, predictive analytics would suggest similar products they should be interested in, therefore increasing the chances of repeat purchases. Salesforce reports personalized experience as an expected outcome of a trader’s business to increase sales by about 20%.
5. Dynamic Pricing
Using predictive analytics, retailers can now execute dynamic pricing strategies that fluctuate prices according to data that is received in real time. For example, adjusting the prices according to demand, competitive pricing, and broader trends in the market could very well be recommended by AI.
This will price optimally the products, allowing them to be competitive and maximize revenue. A study by Deloitte indicates that dynamic pricing strategies can potentially increase sales by 5% to 10% for retailers.
How Predictive Analytics Improves Retail Operations
1. Improved Customer Experience
Through predictive analytics, customers are better placed to enjoy their shopping experience. In this regard, predictive analysis provides a means to interpret customer data and present more personalized offers, item suggestions, and even targeted marketing messages. Such service might easily assist a customer in finding what he/she needs, at the time that he/she needs it.
As an example, predictive models can help suggest items that a customer is most likely to buy based on previous purchases made within a defined timeframe. It can generate higher conversion rates and improve customer satisfaction.
2. Reduced Operational Costs
Predictive analytics makes it possible for retailers to determine the quantity of inventory to keep and thereby save on the wastage of excess stock. Accurate demand predictions help businesses stock only the items that they need and avoid too much inventory and costly storage costs.
Additionally, they can optimize labor costs according to predicted peak buying hours and can help cut down expenses without compromising manpower when understaffed during non-peak hours.
3. Better Decision-Making
Predictive analytics shifts discussion in retail from intuition or gut feeling to good decision-making based on evidence. AI-backed insights may guide businesses for real-time strategy changes, be it through price change, stock optimization, or marketing campaign planning, thereby making retail decision-making more robust and agile in the market.
4. Supply Chain Optimization
One of the most important effects that predictive analytics will have is supply chain optimization. Changes that would have happened, such as fluctuations in demand, disruption in the supply chain, and customer preference change, will be anticipated by predictively adjusting their supply chain strategies on the part of the retailers. This will avoid production delays and will guarantee the availability of products with minimized excess stock.
Additional Benefits of Predictive Analytics in Retail
In addition, predictive analytics helps retailers refine stock, pricing, and promotions. For example, by analyzing customer behavior, they can predict stockouts, thus minimizing lost sales. Moreover, it reveals price elasticity, allowing price adjustments for optimal revenue. Similarly, it forecasts seasonal demand, enabling stock optimization, thus avoiding overstock. Furthermore, predictive tools detect shifts in behavior, enabling quick strategic adjustments. In turn, retailers can align marketing, products, and promotions with demand. As a result, risks decrease, and new opportunities emerge. Ultimately, predictive analytics enhances agility, ensuring success in a dynamic market.
WebMob Technologies: Helping Retailers Harness Predictive Analytics
At WebMob Technologies, we specialize in helping businesses leverage AI/ML development services to integrate predictive analytics into their retail operations. With over 14 years of experience in AI and machine learning, our team can help your retail business. We predict customer demand, optimize inventory and improve sales forecasting through custom AI solutions.
Our custom AI/ML models are tailored to your business needs. This ensures you get the most accurate predictions and insights. Whether it’s retail forecasting or inventory optimization, we help you navigate the complex retail landscape with AI-powered solutions.
If you’re ready to take your retail operations to the next level, contact WebMob Technologies today for a consultation.
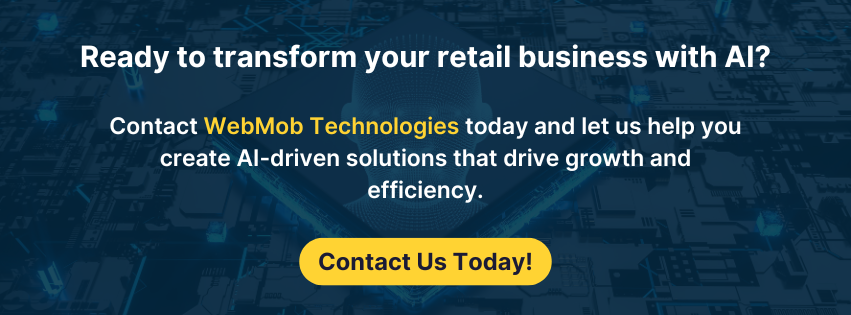
Real-World Examples of Predictive Analytics in Retail
1. Supply Chain Management
Predictive analytics can identify potential supply chain disruptions before they occur. For example, if there is a delay in raw materials, predictive tools can help businesses adjust stock levels accordingly. In addition, these tools can identify alternative suppliers, therefore preventing disruptions. As a result, retailers using these solutions are better prepared to handle unexpected events, keeping their shelves stocked and customers satisfied. Ultimately, predictive analytics ensures a more resilient and responsive supply chain.
2. Customer Segmentation
Retailers can use predictive analytics to divide customers into different segments based on their purchasing habits and preferences. As a result, this enables targeted marketing campaigns, personalized offers, and optimized product recommendations. Moreover, these tailored approaches improve customer engagement and increase conversion rates. In turn, businesses can build stronger relationships with customers, leading to higher loyalty and repeat purchases.
3. Store Location and Merchandising Strategy
Using predictive analytics, retailers can determine the best locations for new stores based on customer demand predictions. Additionally, businesses can optimize their in-store layout by predicting which products are likely to perform well. This helps place products in specific areas of the store, therefore driving better sales.
Conclusion: Predictive Analytics is Shaping the Future of Retail
The power of predictive analytics in retail is undeniable. By leveraging AI and machine learning, retailers can forecast demand, optimize inventory, and personalize customer experiences. As a result, they streamline operations, reduce waste, and improve profitability. Moreover, real-time insights and data-driven decision-making enable businesses to stay ahead of the competition. Consequently, they can also deliver a superior shopping experience to their customers, thereby driving loyalty and increasing sales.
At WebMob Technologies, we help businesses harness the power of predictive analytics. With our AI/ML development services, we offer tailored AI-powered solutions that improve forecasting, inventory management, and overall retail operations.