How AI is Powering the Future of Autonomous Vehicles
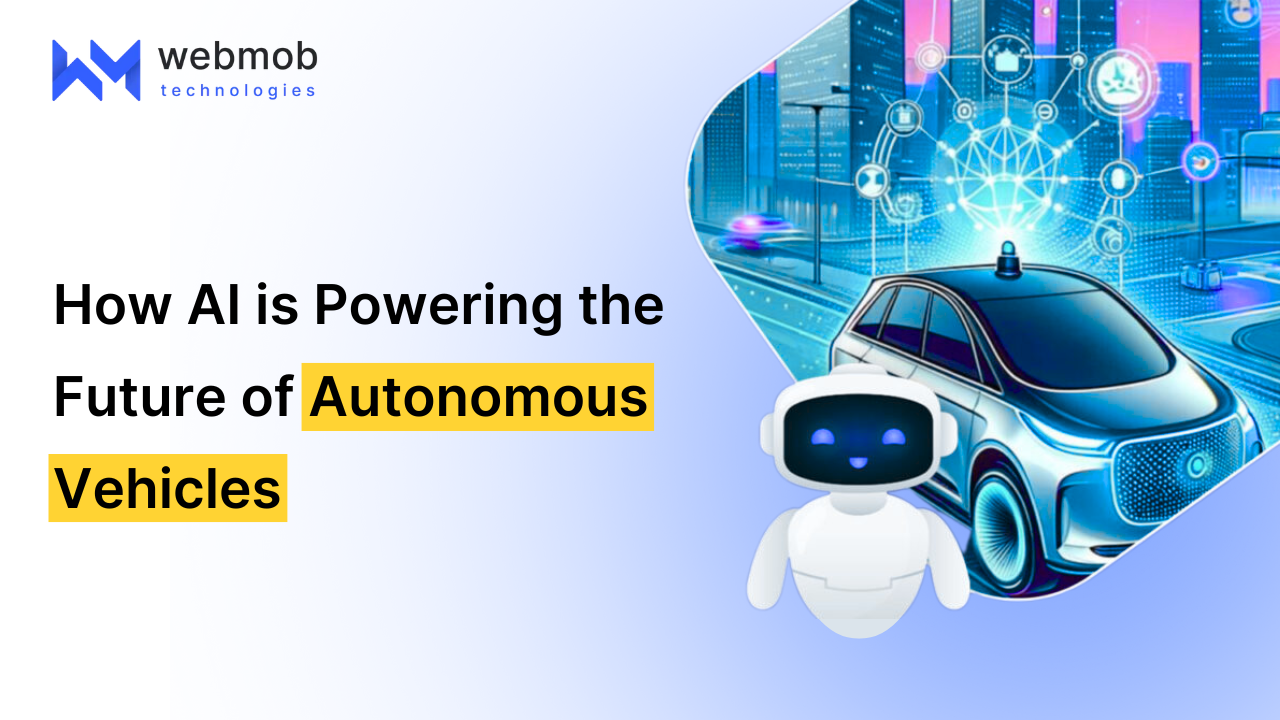
Thinking about a world with fewer accidents on the road, where traffic jams aren’t quite the headache they are today, and getting around is just plain easier for everyone? Honestly, that vision feels like it’s getting closer every day, and it’s largely thanks to autonomous vehicles, or AVs as you often hear them called. You know, human error is, perhaps unsurprisingly, behind a huge number of road accidents – you see the stats, they’re pretty eye-opening. So, the idea of automated driving solutions isn’t just a cool futuristic concept anymore, it feels pretty necessary. And really, Artificial Intelligence, or AI, isn’t just helping autonomous vehicles along; it’s fundamentally the engine making this whole self-driving revolution possible. Just look at reports, like the one from McKinsey, they talk about how AI is absolutely key to unlocking the potential here.
So, in this post, we want to dive a bit deeper into how and why AI is so central to autonomous vehicles. We’ll explore some of the core technologies that make it all work and, naturally, touch on some of the big challenges that still need sorting out. It’s all about seeing how AI is really shaping the future of how we get from point A to point B, focusing specifically on the connection between AI and these autonomous vehicles, and the specific tech that’s bringing it to life.
Understanding the Foundation: What are Autonomous Vehicles?
Autonomous vehicles, or self-driving cars if you prefer – the terms are used pretty interchangeably – represent, you could say, a really big shift in how we think about transportation. Essentially, they use a bunch of sensors, along with clever software and AI, to find their way and operate without needing a human driver to do the hands-on work.
Defining Autonomous Vehicles and Their Evolution
The Society of Automotive Engineers, or SAE, came up with this useful way to categorize just how automated a vehicle is. There are six levels, running all the way from Level 0, which is basically zero automation, right up to Level 5, where the car handles absolutely everything. Understanding these levels is, well, it’s pretty important for grasping where we are now and where things are headed with autonomous driving.
The Levels of Driving Automation (SAE J3016 Explained)
It starts simply enough, you know?
- Level 0: This is your standard car today. The driver is in complete control, doing all the steering, braking, accelerating.
- Level 1: You start seeing some help here, driver assistance. Think cruise control or systems that help you stay in your lane – they assist, but you’re still doing most of the driving.
- Level 2: This is Partial Automation. It combines things like adaptive cruise control with lane centering. The car can handle steering and speed together in certain situations, but you absolutely have to stay paying attention and be ready to take over. It’s still on you.
- Level 3: Conditional Automation is where it gets interesting. The vehicle can actually handle some driving tasks on its own under specific conditions, like on a highway in clear weather. But, and this is a big but, the driver still needs to be ready to jump back in when the system asks them to.
- Level 4: High Automation means the vehicle can handle all driving tasks, but only within specific, defined conditions or areas – maybe a particular city or a designated highway route. In those areas, you don’t need to be ready to intervene; the car should handle it.
- Level 5: This is the ultimate goal, Full Automation. The vehicle can handle every driving task in literally all conditions, everywhere a human could drive. No human driver is needed at all.
Why the Distinction is Important
These levels really help show that this isn’t an overnight switch; it’s a gradual journey towards full autonomy. They clarify, for example, who is responsible – is it the person in the seat, or is the vehicle taking over entirely? It clears things up, which is good.
The Essential Components of an Autonomous Vehicle
Think about what makes up one of these cars. It’s a pretty complex setup, a system with several key parts all needing to work together seamlessly.
Sensory Systems (Cameras, LiDAR, Radar, etc.)
These are, you could say, the vehicle’s eyes and ears. They’re constantly collecting data about what’s going on around the car. You’ve probably heard of some of them:
- Cameras: These give the vehicle visual information, like recognizing colors, signs, textures.
- LiDAR: This uses lasers to create a detailed 3D map of the surroundings. It’s great for precise measurements.
- Radar: This uses radio waves and is good at detecting objects and their speed, especially useful in bad weather where cameras or LiDAR might struggle.
- Ultrasound: This is usually for very short-range detection, like when you’re parking, helping detect nearby obstacles.
High-Performance Computing Platforms
All that sensor data has to go somewhere and be processed, and fast. This requires really powerful computers inside the car that can run the AI algorithms. These systems need a lot of processing grunt, obviously, but also need to be pretty energy efficient, which is, you know, a technical challenge.
Sophisticated Software Stack
Underneath it all is the software. This includes all the AI algorithms that handle things like figuring out what’s around the car (perception), deciding what to do next (planning), and then actually doing it (control). There’s also the operating system and how everything communicates. It’s a layered system, a ‘stack’.
Why AI is the Only Viable Path to True Autonomy
So, why do we need AI for this? Why can’t we just, I don’t know, program a car to follow a really complicated set of rules?
Moving Beyond Traditional Rule-Based Systems
Traditional systems basically work by having pre-programmed instructions for specific situations. If you see X, do Y.
The Inadequacy of Pre-programmed Responses to Infinite Scenarios
The problem is, the real world isn’t neat and tidy. It’s practically impossible, or maybe just completely impossible, to think of and program for every single driving situation you might encounter. Traffic, weather, unexpected events – the real world is just too complex and unpredictable for simple “if-then” rules to cover everything safely.
The Need for Flexibility and Adaptability
Self-driving cars absolutely have to be able to adjust to changing conditions and handle things they weren’t specifically programmed for. This is where AI comes in, because it has that ability to learn and generalize from data, which is something traditional systems just can’t do.
AI’s Ability to Handle Complexity and Uncertainty
AI, especially techniques like machine learning, is really good at taking in vast amounts of information and making decisions even when things aren’t perfectly clear or certain.
Processing Massive Amounts of Real-time Data
Think about how much data an autonomous vehicle’s sensors are collecting every second – it’s a huge amount, like terabytes per day. AI algorithms are essential for processing all this data efficiently so the car can understand its surroundings in real time.
Navigating Unforeseen ‘Edge Cases’ in the Real World
You hear the term “edge cases” a lot in this field. These are those rare, unusual, weird scenarios that traditional systems just can’t cope with because they weren’t designed for them. AI, through learning from massive datasets and simulations, has a much better chance of learning how to handle these unexpected situations. It’s not perfect, mind you, but it’s significantly better equipped.
Learning and Continuous Improvement Through Data
One of the really powerful things about AI is that its performance can get better over time just by being exposed to more data. This constant learning loop is absolutely critical for making autonomous driving safer and more reliable the more it’s used and tested.
The AI Powerhouse: Key AI Techniques Enabling Autonomous Driving
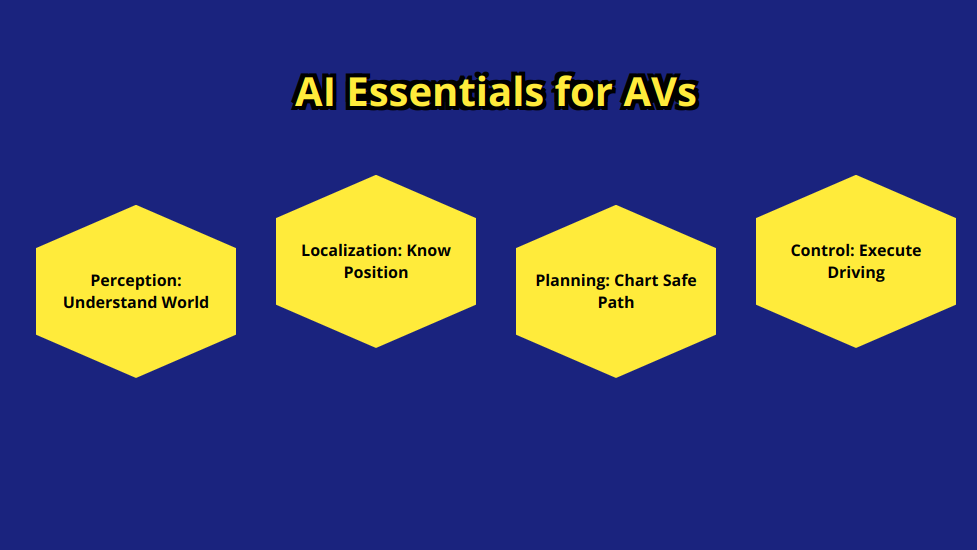
Let’s break down some of the specific ways AI is used within an autonomous vehicle system.
Perception: Giving the Autonomous Vehicle ‘Eyes’ and ‘Ears’
This is all about the car understanding what’s around it based on the sensor data. This is really where machine learning in transport starts to show its stuff.
Sensor Fusion: Integrating Data from Disparate Sensors
Since no single sensor is perfect, the car needs to combine information from all of them. This is called sensor fusion. It’s like using multiple senses together to get a better picture of the world.
How Cameras, LiDAR, Radar, and Ultrasound Complement Each Other
Think of it this way:
- Cameras: These see colors and details, like traffic signs.
- LiDAR: This gives very precise distance and shape information in 3D.
- Radar: This uses radio waves and is good at detecting objects and their speed, especially useful in bad weather where cameras or LiDAR might struggle.
- Ultrasound: This is usually for very short-range detection, like when you’re parking, helping detect nearby obstacles.
They all provide different pieces of the puzzle, and you need to combine them.
Algorithms for Fusing Data Streams
There are specific algorithms, like Kalman filters or Bayesian networks, used to mix all this sensor data together. The goal is to reduce noise and inaccuracies from individual sensors to get the most accurate understanding of the environment possible.
Object Detection and Recognition with Deep Learning
Once you have the data, the car needs to figure out what the objects are. This is where deep learning algorithms come in, identifying and classifying things.
Convolutional Neural Networks (CNNs) Explained
A particularly important type here is Convolutional Neural Networks, or CNNs. They are really well-suited for processing image data. They essentially learn to pick out features in images – shapes, edges, patterns – and then use those features to figure out what the object is, sorting them into different categories.
Identifying Vehicles, Pedestrians, Cyclists, Animals, and Static Objects
Using CNNs, the car can identify a huge variety of things around it: other cars, people walking, bikes, even animals, or things that aren’t moving like barriers or signs. This is fundamental to knowing what the car needs to interact with or avoid.
Training Data Requirements and Challenges
Training these CNNs is a massive undertaking. It requires absolutely huge amounts of labeled data – images and videos where someone has already gone through and marked everything: “that’s a pedestrian,” “that’s a traffic light,” “that’s the road.” Getting enough data that covers all sorts of different conditions and situations is a major challenge.
Semantic Segmentation: Understanding the Scene’s Layout
Beyond just identifying objects, the car also needs to understand the layout of the scene. Semantic segmentation helps with this by classifying every single pixel in an image.
Identifying Road Surfaces, Sidewalks, Buildings, Vegetation at a Pixel Level
This means the car can tell, pixel by pixel, what is the actual road surface it can drive on, what is the sidewalk, what’s a building, what are trees, and so on. It helps the car understand where it can go and where it can’t.
Instance Segmentation (Distinguishing individual objects of the same class)
And it gets even more granular with instance segmentation. This lets the car tell the difference between individual objects of the same type – for example, not just knowing “there are pedestrians here,” but knowing “that is one pedestrian, and that is a separate pedestrian next to them.”
Tracking and Predicting Object Behavior Over Time
It’s not enough to just see objects; the car needs to keep track of them as they move and, crucially, try to predict what they’re going to do next.
Multi-Object Tracking (MOT) algorithms
Algorithms called Multi-Object Tracking help the car maintain a consistent identity for objects over time. Even if an object gets temporarily hidden behind something or moves out of sight for a moment, the system tries to keep track of it.
Using AI to Predict Intent and Trajectories of Other Agents
This is a really tricky but vital part. Can AI learn to predict, for example, if that pedestrian is about to step off the curb? Or if the car in the next lane is planning to merge? AI can analyze patterns in how other road users behave and try to anticipate their actions, which is obviously critical for safe planning.
Localization and Mapping: Knowing ‘Where’ the Vehicle Is
The car needs to know its precise location in the world (localization) and have a representation of its environment (mapping).
Global Positioning Systems (GPS) Limitations
You might think GPS is enough, but it’s actually not accurate enough for self-driving cars, especially in cities where buildings block signals. It can also be unreliable, like in tunnels or under bridges.
Simultaneous Localization and Mapping (SLAM)
SLAM algorithms are pretty clever – they let the vehicle build a map of its surroundings while simultaneously figuring out where it is within that newly built map.
The Role of High-Definition (HD) Maps and how AI uses them
HD maps are incredibly detailed and accurate maps that provide much more information than standard GPS maps – things like lane lines down to a few centimeters, road boundaries, even the height of curbs. AI algorithms use these HD maps as a reference point to significantly improve their localization accuracy and help with planning maneuvers.
Decision Making and Planning: The Autonomous Brain’s Strategy
Once the car understands its environment, it has to decide what to do and plan how to do it.
Path Planning: Charting the Course Safely and Efficiently
This is about figuring out the best way to get from point A to point B while avoiding anything in the way.
Global Path Planning (Route from A to B)
This is like your sat-nav planning the overall route for a long journey, taking into account traffic, road closures, etc.
Local Motion Planning (Navigating Immediate Obstacles)
This is more immediate – if a ball rolls into the street or a car stops unexpectedly, the local planning adjusts the vehicle’s path right then and there to safely go around it.
Algorithms like A, RRT, and Reinforcement Learning approaches
Various algorithms are used for this, from classic search algorithms like A or RRT (Rapidly-exploring Random Tree) to more modern approaches using reinforcement learning, where the system learns the best actions through trial and error (in simulations, mostly!).
Behavior Planning: Deciding ‘What’ to Do in Complex Scenarios
Beyond just following a path, the car needs to make higher-level decisions about how to behave in complex traffic situations.
Lane changes, merging, turning, reacting to traffic signals
This involves figuring out the right time and the safest way to change lanes, merge onto a highway, make a turn at an intersection, or react correctly to traffic lights and stop signs.
Using Machine Learning to imitate human-like decision-making
Interestingly, researchers are using machine learning to train vehicles to make decisions that feel more natural, more like a human driver would in tricky situations. It’s about learning the nuances of traffic flow.
Handling Uncertainty and Risk Assessment in Planning
Any planning system for a car has to deal with uncertainty. The world isn’t perfectly known. Planning algorithms need to consider potential risks associated with different actions – is it safe to merge now, or is there too much uncertainty about that approaching car’s speed?
Control: Translating Decisions into Physical Actions
Okay, so the car has perceived the world and planned its actions. Now, it actually has to do it. This is the control part – making the steering wheel turn, hitting the accelerator or brake pedal.
Executing the Plan: Steering, Acceleration, and Braking
This requires really precise control over the vehicle’s physical components that make it move and stop.
Control algorithms (PID, Model Predictive Control – MPC)
Specific control algorithms, like PID controllers or Model Predictive Control (MPC), are used here to ensure the car executes the planned movements accurately and smoothly.
Ensuring Smooth and Safe Vehicle Dynamics
The control system is also responsible for making sure the car behaves in a stable and safe way. You don’t want jerky steering or sudden braking unless absolutely necessary. It’s about keeping the ride smooth and the vehicle under control.
Machine Learning in Transport: Beyond the Driving Stack
It’s worth mentioning that AI and machine learning applications aren’t just limited to the actual driving part.
Predictive Maintenance using ML for Fleet Management
For companies managing fleets of autonomous vehicles, machine learning can analyze data to predict when certain components might be about to fail. This allows for proactive maintenance, fixing things before they break down, which saves money and keeps the vehicles running.
Optimizing Traffic Flow with AI and AV Integration
Looking ahead, as more AVs are on the road, AI could potentially optimize traffic flow across entire networks by coordinating how these vehicles move, perhaps reducing congestion for everyone.
The Importance of Simulation for Training and Validation
You can’t test everything on public roads right away, obviously. Simulation is absolutely essential. It allows developers to train AI algorithms on millions or billions of miles of virtual driving scenarios, including those rare edge cases, and validate that the system works correctly in a safe, controlled environment before ever putting it in a real car on a real street.
The Road Ahead: Challenges AI Must Overcome
So, it’s clearly powerful, but it’s definitely not a done deal. There are some pretty big hurdles still.
The ‘Long Tail’ Problem: Rare Events and Adversarial Conditions
People often talk about the “long tail” problem. It refers to the challenge of handling those extremely rare, unusual events that don’t happen very often but can still be dangerous.
Dealing with Snow, Heavy Rain, Unseen Obstacles
Things like driving in a blinding snowstorm, navigating heavily flooded streets, or encountering unexpected debris in the road are still really tough for AI to handle reliably compared to a human driver with years of varied experience.
Data Requirements for Exceedingly Rare Scenarios
Training AI to handle these rare situations requires, perhaps counterintuitively, a lot of data specifically from those rare scenarios, which are, well, rare! It’s a bit of a Catch-22.
Ensuring AI Safety, Reliability, and Explainability
This is probably the most critical challenge. How do we know the AI is truly safe and reliable in all possible situations? And can we even understand why it made a certain decision if something goes wrong?
The Black Box Problem: Understanding AI Decisions
AI systems, especially deep learning ones, can sometimes feel like a “black box.” They give you an answer, but it’s incredibly difficult, sometimes impossible, to trace back exactly why the system came to that particular conclusion. This makes troubleshooting and building trust tricky.
Verification and Validation of Complex AI Systems
Proving, rigorously, that a complex AI system is safe and reliable in all operating conditions is a huge task. It’s not like traditional software where you can test every line of code or every rule. These systems learn and adapt.
Cybersecurity Threats to AI in AVs
Given how connected these vehicles are and how central the AI is, they are unfortunately potential targets for cyberattacks. Ensuring the AI is secure and cannot be tampered with is paramount.
Computational Demands and Energy Efficiency
Running all these complex AI algorithms requires a lot of computing power. Fitting that into a car, making it energy efficient so it doesn’t drain the battery too quickly (especially for electric vehicles), is a significant engineering challenge.
Regulatory Hurdles and Public Acceptance
Beyond the tech, there are also non-technical challenges. Getting the right regulations in place to govern these vehicles is complex, and perhaps even more importantly, getting the public to trust and accept autonomous vehicles is vital for widespread adoption. People need to feel safe riding in and being around them.
Building Trust: Safety Frameworks and Testing
So, how are developers trying to address the safety concerns and build that trust?
Redundancy in Hardware and Software
Building safety into the system involves redundancy. This means having backup systems, both in the physical hardware and the software, so if one part fails, another can take over to ensure the vehicle can still operate safely, or at least come to a safe stop.
The Role of Simulation and Real-World Testing
As mentioned before, simulation is crucial for testing millions of scenarios, but real-world testing is absolutely irreplaceable. Driving actual miles in diverse conditions helps uncover issues that simulations might miss. Both are essential parts of the development process.
Industry Standards and Certification Processes
Like any critical technology, there’s a push for industry-wide standards and certification processes. These help ensure that autonomous vehicles meet certain agreed-upon safety requirements and benchmarks before they’re allowed on the road.
Human-Machine Interface (HMI) Design for Safety
The interface between the vehicle and any human occupants (or remote operators) is really important too. The HMI needs to be clear, intuitive, and provide the human with just the right amount of information about what the vehicle is doing, what it plans to do, and any limitations or situations where the human might need to pay attention or take over (in lower automation levels).
The Broader Impact: Ethical and Societal Considerations
It’s also important to think about the bigger picture. How will autonomous vehicles change society?
Ethical Dilemmas: The ‘Trolley Problem’ and Responsibility
This is a heavy one, the famous “trolley problem” kind of scenario. In an unavoidable accident situation, if the car has to make a choice between two bad outcomes – say, hitting obstacle A or obstacle B – how is that decision programmed? Who decides the ethical framework? And ultimately, who is responsible when something goes wrong? These are really complex questions.
Impact on Employment and the Future of Transportation Jobs
It’s pretty clear that widespread autonomous vehicle adoption will impact jobs, particularly for professional drivers – truck drivers, taxi drivers, delivery drivers. How society manages this transition and supports those affected is a significant challenge.
Accessibility and Inclusivity for People with Disabilities
On a more positive note, autonomous vehicles hold incredible potential to improve mobility and independence for people with disabilities or the elderly who might currently struggle to drive or access transportation. That’s a huge potential benefit.
Reshaping Urban Infrastructure and Planning
Think about how cities are designed now – they’re built around human-driven cars. As AVs become more common, it could change everything from parking needs to traffic management systems to the very layout of streets and intersections. It could lead to more efficient and perhaps greener urban environments, but it requires rethinking a lot of existing infrastructure.
The Future Landscape: Emerging Trends in AI and AVs
Where is this all headed?
Vehicle-to-Everything (V2X) Communication and Collective Intelligence
The idea here is for vehicles to not just sense their immediate surroundings, but to talk to each other, to the traffic lights and infrastructure, and even to pedestrians’ phones. This V2X communication could create a kind of collective intelligence on the road, allowing vehicles to anticipate problems much earlier and coordinate movements for smoother traffic.
Advancements in AI Hardware (Edge AI, Specialized Processors)
Processing all that data in real-time inside the car (at the “edge”) is getting a boost from new, more powerful and efficient computer chips designed specifically for AI tasks. This “Edge AI” is key to improving performance without needing huge power consumption.
The Role of 5G and Beyond in Enabling Connectivity
Future wireless technologies, like 5G and whatever comes after, are crucial because they offer the high speed and low delay needed for vehicles to communicate with each other and the infrastructure instantly. This is essential for things like V2X communication and relying on cloud-based AI updates or processing.
Integration with Smart Cities and IoT Ecosystems
Autonomous vehicles won’t exist in isolation. They’ll likely become integrated parts of larger smart city ecosystems, talking to smart traffic lights, coordinating with public transport, and potentially offering new services we haven’t even thought of yet as part of the Internet of Things.
The Ongoing Journey Towards Full Level 5 Autonomy
Getting to full Level 5 autonomy everywhere is, let’s be honest, still a long-term goal. It’s an ongoing journey that requires constant innovation, testing, and a lot of collaboration across different industries and even governments.
Partnering in Innovation: How WebMob Technologies Drives the Future of Autonomous Tech.
This kind of transformative technology needs serious expertise. WebMob Technologies, for example, has experience modernizing industries, bringing their skills in things like AI, Machine Learning, and general software development to the table.
WebMob’s Expertise in AI, Machine Learning, and Software Development.
They focus on using modern methods and building solutions that are, you know, robust, reliable, and can scale up as needed for businesses in the automotive and transportation space.
Building Robust, Reliable, and Scalable Solutions for Automotive and Transportation.
Essentially, they’re ready to work with companies to help develop the next generation of autonomous capabilities, turning these possibilities into actual working systems.
It really is about partnership and bringing specialized skills together to push this technology forward.
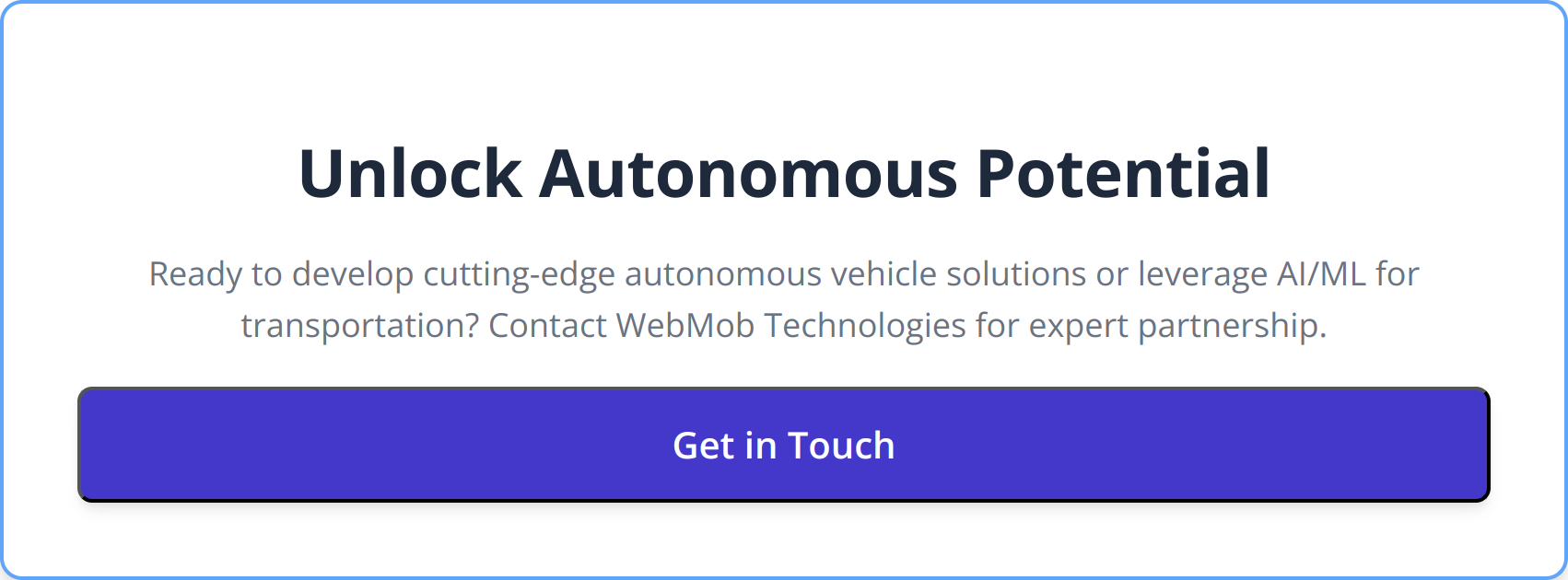
Conclusion
So, wrapping things up, it’s pretty clear that AI isn’t just part of the autonomous vehicle story – it’s really the driving force behind this whole revolution, pun intended. From helping the car understand the world around it (perception) to figuring out where to go (planning) and then making it happen physically (control), AI is enabling vehicles to navigate safely and, hopefully, more efficiently than human drivers in the long run. There are definitely challenges still, big ones, and we talked about a few of them. But the potential benefits of autonomous vehicle tech are honestly huge – think about the safety gains alone. Getting there is going to require a lot of ongoing innovation and, crucially, collaboration across the industry.
Discover the Difference
Interested in exploring how AI, ML, and custom software can benefit your business, maybe even in the autonomous space? WebMob Technologies offers services tailored to developing this kind of cutting-edge technology.
Feel free to reach out to WebMob for expert consultation if you’re looking to develop autonomous vehicle solutions or similar tech.
And hey, if you found this interesting and want more insights into new technologies, consider subscribing to the WebMob blog!
FAQs
- What are the main benefits of autonomous vehicles? People usually highlight things like fewer accidents, hopefully less traffic congestion, and potentially making transportation much easier for people who can’t drive themselves.
- What are the biggest challenges facing autonomous vehicle development? Well, there are quite a few, but definitely ensuring they are truly safe in every conceivable situation is number one. Handling those really rare, tricky events is tough, and then there are the ethical questions that come up.
- When will fully autonomous vehicles be widely available? That’s a tough one to put an exact date on, to be honest. Experts are guessing maybe sometime in the next decade, but there are still a lot of hurdles to clear first.
- How are autonomous vehicles tested for safety? It’s a mix of things. They do a lot of testing in simulations, which is crucial, but also extensive real-world testing on roads. And they have to meet certain industry standards and go through certification processes.
- What is the role of AI in autonomous vehicle safety? AI is fundamental to the car even being able to drive itself. It helps the car sense everything around it, plan its route safely, and control the steering and speed. All of that AI-powered capability is what’s intended to make the roads safer by eventually reducing human error.