The Future of Manufacturing: How AI is Optimizing Operations and Reducing Costs
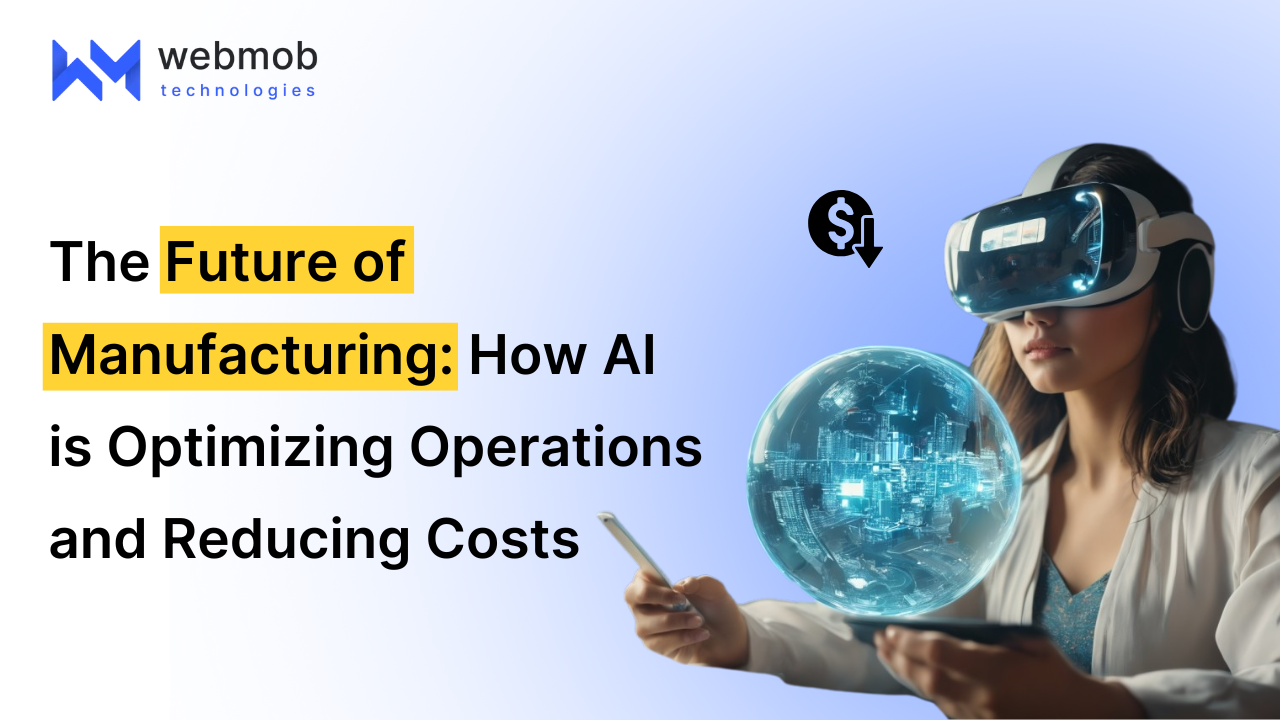
The manufacturing industry, well, it truly feels like it’s standing at a really interesting point right now, perhaps even a critical one. It seems poised for a transformation that could be pretty unprecedented. All over the world, you see factories evolving, and a lot of that change is definitely being driven by technology. These advancements promise, and often deliver, big jumps in efficiency and, of course, reductions in costs.
Artificial Intelligence, or AI, isn’t some far-off concept anymore; it’s become a real driving force behind this shift. It’s fundamentally reshaping how manufacturing operations work, from making production lines smoother to spotting potential equipment problems before they happen. This really does lead to enhanced efficiency and, yes, significant cost savings. The old headaches manufacturing used to face – things like machines suddenly breaking down, quality issues creeping in, or supply chains just not being as smooth as you’d like – AI-powered solutions are now taking these on directly. You hear estimates, like from McKinsey, suggesting AI could potentially add a huge amount, maybe even $3.7 trillion, to the global manufacturing economy by 2030. That’s… well, that’s a lot.
So, in this post, I want to dive a bit into the main ways AI is being used in manufacturing, talk about the good stuff – the benefits – but also acknowledge the challenges, and then maybe peer into what the future might hold. I think exploring this is essential if companies want to stay competitive down the line. We’ll look at how AI is, in a way, paving the path for smarter factories and ushering in what feels like a new era of manufacturing excellence.
Understanding the AI Landscape in Modern Manufacturing
Alright, so when we talk about AI in manufacturing, what exactly are we referring to? It’s really an umbrella term covering a bunch of different technologies. This includes things you’ve probably heard of like Machine Learning (ML), Deep Learning, Computer Vision, and Natural Language Processing (NLP). Think of it this way: machine learning is about systems learning from data. Deep learning uses these really complex structures, like neural networks, to find advanced patterns. Computer vision lets machines actually “see” and figure out what’s in images, and NLP… well, that helps machines understand and process human language. These aren’t just theoretical tools; they’re being applied right onto the factory floor in various processes.
Now, you might wonder why AI adoption seems to be accelerating so quickly. There are a few reasons, I think. For one, we just have so much more data available now, especially from all those IoT devices scattered around a plant. Plus, computing power is way more accessible and affordable than it used to be. The AI algorithms themselves are also getting better, more mature. And, let’s be honest, businesses today just need to be more adaptable and resilient than ever before. How does AI differ from, say, traditional automation? Well, traditional automation typically just follows instructions that someone programmed in beforehand. AI, though, can actually learn and adapt based on new data it receives, allowing it to optimize things pretty much in real-time. That’s a key difference.
Core AI Applications Transforming Manufacturing Operations
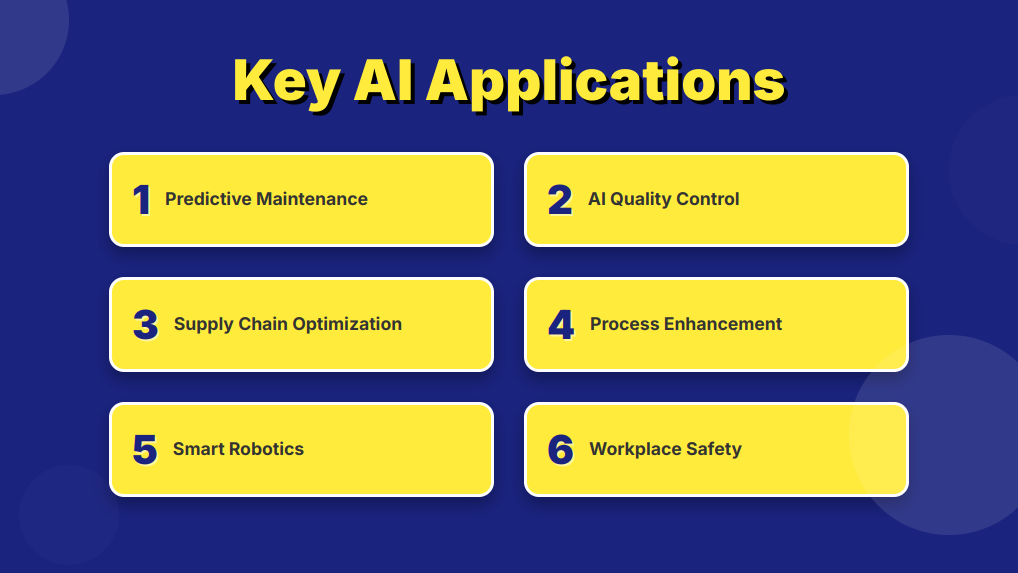
Let’s look at some of the practical ways AI is showing up and making a difference.
Take Predictive Maintenance, for example. This is a big one. It uses data from sensors, historical records, and machine learning to predict when a piece of equipment might fail before it actually gives out. It’s a proactive approach, you see, which really helps keep things running smoothly and avoids those incredibly costly unexpected stops. How does it generally work? Well, sensors on machines gather real-time data – temperature, vibration, pressure, all that sort of thing. Machine learning models then chew through this data, looking for patterns that suggest trouble is brewing. When something looks off, alerts get sent out, and maintenance can be scheduled ahead of time.
The benefits here are clear: less unplanned downtime, equipment potentially lasting longer, maintenance schedules getting smarter, and overall maintenance costs often dropping. It usually improves what’s called OEE, or overall equipment effectiveness. Imagine a beverage company, for instance. They might use this on their bottling machines. If sensors pick up weird vibrations, the AI system could flag a potential bearing failure. They schedule the fix before the machine breaks down entirely, preventing a whole production line stoppage and saving, I’d guess, thousands of dollars in lost production.
Then there’s AI-Powered Quality Control. This is another area where AI, particularly computer vision and deep learning along with sensors, is making a huge impact. It helps with inspecting products and finding defects right there on the line, aiming for really flawless production. Basically, cameras snap pictures or videos of products as they move along. Deep learning algorithms are trained to recognize what a good product looks like and identify deviations – those scratches, dents, or assembly errors based on visual patterns. The system flags anything that looks wrong, anything that deviates from the norm. Sometimes, automated systems can even remove those defective products from the line automatically.
This means more consistent product quality, less waste and rework, faster inspection cycles than a human could manage, and it can even help spot process issues early on because it’s catching quality problems quickly. Think about detecting tiny scratches on a finished surface, making sure every single screw is in place during assembly, or just verifying that a part is the right size.
AI is also doing some fascinating things with Optimizing the Supply Chain. It’s being used to get a better handle on forecasting demand, managing inventory levels more smartly, improving logistics, and even helping with how companies work with their suppliers. Machine learning models are key here, predicting future demand by looking at past sales, market trends, and other outside factors. Then, other optimization algorithms jump in to figure out the best routes for shipping or the ideal amount of inventory to keep on hand. The system can even spot weird anomalies in logistics data. All of this usually adds up to lower inventory costs, products getting delivered on time more consistently, reduced shipping expenses, a better ability to react to market changes, and generally a more resilient supply chain.
Enhancing Production Processes themselves is another major application. AI can analyze tons of process data – things like temperature, pressure, speed settings – and figure out how they relate to outcomes like how much product you get (yield) or how much energy is being used. It can then recommend or even automatically tweak those settings in real-time to get better results. The benefits? Often, higher production output, less energy consumed, less waste generated, resources being used more effectively, and just a more stable, predictable process overall. I remember hearing about an aluminum smelting plant, for instance, using AI to optimize furnace temperature based on data from multiple sensors. Apparently, it led to a pretty significant reduction in energy consumption – something like 15%.
And let’s not forget about Smart Robotics. AI is definitely changing the game for robots on the factory floor, making them more flexible, able to adapt, and even collaborate safely with people. We’re talking about robots that use computer vision to actually “see” and understand their surroundings, robots that can learn new tasks with AI, and collaborative robots (cobots) that are designed to work right alongside human colleagues safely. They can even navigate complex factory layouts autonomously. This means manufacturing becomes more flexible, robots can handle trickier tasks, safety improves in shared workspaces, and overall efficiency gets a boost.
Finally, AI is even helping with Improving Workplace Safety. It can monitor safety procedures, identify potential hazards, and try to predict risks before accidents happen. This might involve computer vision systems checking if workers are wearing the right safety gear (PPE), analyzing spatial data to spot risky areas, monitoring environmental conditions, or even identifying potentially unsafe actions workers might be taking. The payoff here is huge: fewer accidents and injuries, a more proactive approach to mitigating risks, better compliance with safety regulations, and ultimately, lower costs associated with workplace incidents.
AI Application | What it broadly does | Some key benefits you often see |
---|---|---|
Predictive Maintenance | Helps predict when equipment might fail using data and learning. | Less downtime, longer equipment life, smarter maintenance. |
AI-Powered Quality Control | Uses vision and learning to inspect products and find defects in real-time. | Better product quality, less scrap/rework, faster checks. |
Supply Chain Optimization | Applies AI to forecast demand, manage stock, and improve logistics. | Lower inventory costs, better on-time delivery, reduced logistics spending. |
Process Enhancement | Analyzes production data to fine-tune settings like temp or pressure. | Higher output, less energy use, less waste. |
Smart Robotics | Makes robots more adaptable, able to learn, and collaborative using AI. | More flexibility, handles complex jobs, improved safety with people. |
Workplace Safety | Monitors safety rules and spots hazards using vision and data analysis. | Fewer accidents, proactive risk management, better compliance. |
Building the Smart Factory of Tomorrow
All these individual AI applications start to converge when we talk about the Smart Factory. This is really a vision of a manufacturing facility that’s highly digital and super connected, using technologies like IoT, cloud computing, and data analytics to become way more efficient and responsive.
Think of AI as sort of the brain in this setup. It’s processing all that data flooding in from IoT sensors on the machines, from the ERP system, from the manufacturing execution system (MES), and everywhere else. Connectivity and data are absolutely crucial here. This means needing IoT sensors everywhere, robust networks (like 5G, which is becoming a big deal here), solid data platforms, and often using edge computing to process some data locally and quickly. Different AI applications don’t just run in silos; they really need to work together, creating a connected, intelligent ecosystem where real-time data is shared across the whole factory. This allows for decisions and adjustments to be made almost instantly, optimizing operations in a way we couldn’t before.
Tangible Benefits: Beyond Efficiency and Cost Savings
While efficiency and saving money are certainly major drivers, the benefits of AI in manufacturing actually go quite a bit deeper.
For instance, AI can really boost a manufacturer’s Agility and Flexibility. It allows companies to react much faster to shifts in market demand or handle unexpected disruptions more smoothly. It also leads to significantly Enhanced Decision Making because insights are based on solid data, not just guesswork. Improved Sustainability is another important outcome; AI helps optimize how resources are used and reduces waste. And, honestly, it often sparks Innovation, leading to entirely new processes or even helping develop new kinds of products.
These benefits combined really give manufacturers a significant competitive advantage. They can respond more effectively to what customers need and operate in a way that’s simply more sustainable in the long run. It’s a pretty compelling picture.
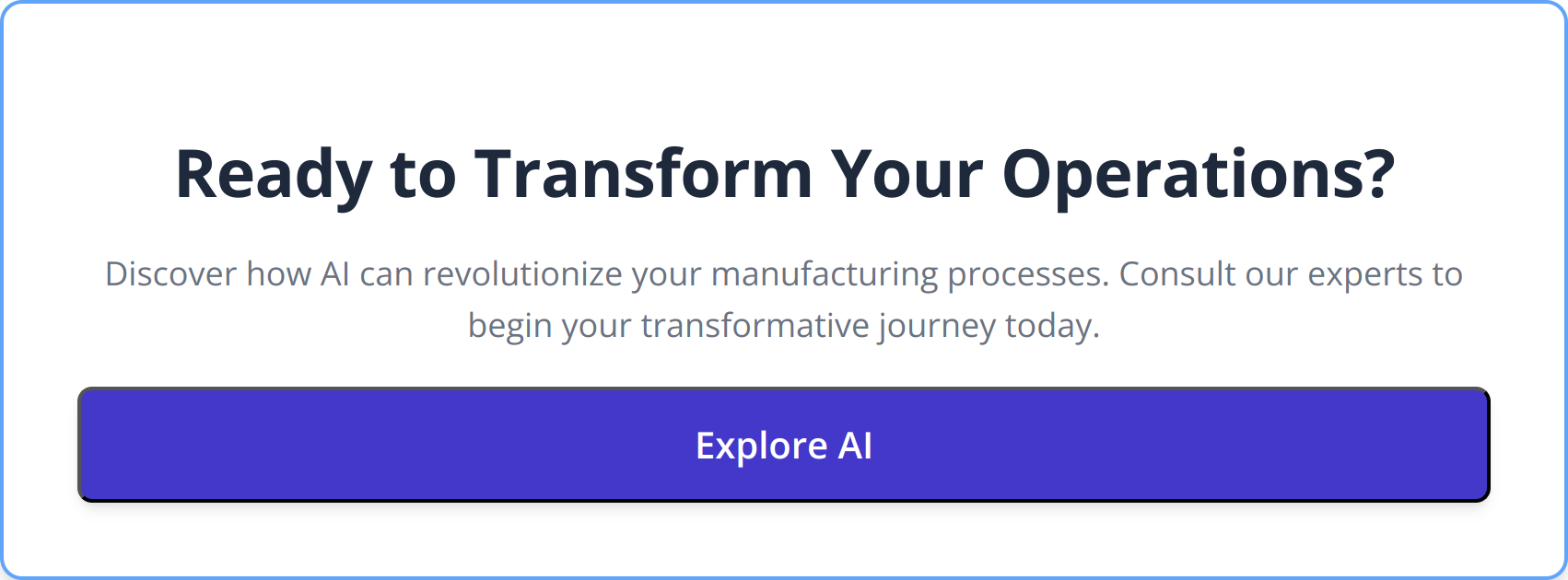
Navigating the Challenges of AI Adoption in Manufacturing
Now, introducing AI into manufacturing isn’t always a walk in the park, of course. There are definitely some challenges you need to be prepared for.
First off, there’s the Data Infrastructure. You absolutely need clean, accessible data for AI to work well, and getting that right can be a project in itself. Then there’s the Talent Gap. You really need a skilled workforce, people who understand how to manage and maintain these AI systems. Integration Complexity is another big one; getting AI to talk nicely with older, legacy systems that might be decades old can be difficult. Security Concerns are paramount – you have to protect your data and your AI models from cyber threats. And, yes, the initial Implementation Costs can be high; it requires investment.
But these challenges aren’t insurmountable. They can be overcome. It often means investing seriously in good data management systems, training your existing employees in AI skills, perhaps starting small with pilot projects to learn the ropes, and definitely putting in place robust security measures right from the start. Making sure your data is secure and preventing unauthorized access is truly key when you’re bringing AI into your operations.
Your Roadmap to Implementing AI: Getting Started
So, if you’re thinking about starting with AI, here are some high-level steps to consider.
First, you probably want to Identify specific pain points in your operation where AI could really make a difference. Don’t try to do everything at once. Then, you need to Assess your data readiness – do you have the data? Is it in a usable format? After that, it’s often smart to Start with pilot projects. Pick one or two areas and try it out. You’ll need to either Build or acquire the right AI expertise, whether that’s hiring new people or working with external partners. Don’t forget to Focus on change management and training for your employees; this is a big shift for people. And finally, Plan for scaling – think about how you’ll expand successful pilot projects across the business.
Implementing AI, you see, is really a journey, not a one-time thing. It’s probably best to start small, learn from what works (and maybe what doesn’t), and then gradually build up your AI capabilities over time.
The Horizon: What’s Next for AI in the Industry?
Looking ahead, there are some really interesting trends that I think will shape the future of AI in manufacturing even further.
We’ll likely see wider adoption of things like Generative AI, more sophisticated ways for humans and AI to work together (going beyond just simple cobots), an increase in overall autonomy within factories, and AI playing a much bigger role in things like the circular economy.
AI is definitely not standing still; it will continue to evolve and, I believe, revolutionize manufacturing processes even more than it already has.
Conclusion: Embracing the AI-Driven Future of Manufacturing
To wrap things up, I think it’s pretty clear that AI is becoming absolutely essential for optimizing manufacturing operations, bringing down costs, and ultimately building those smart factories we talked about.
It really is a transformative force. Manufacturers who want to stay competitive simply have to embrace it. By adopting AI, companies can unlock incredible new levels of efficiency, make their products better, and operate in a more sustainable way. If you’re curious about exploring how AI could specifically transform your manufacturing operations, maybe think about consulting with experts, someone like the folks at WebMob Technologies, for instance.
Ultimately, the future, at least in manufacturing, really does seem to belong to those who are willing to adopt AI. You really don’t want to be left behind, do you?
FAQs
Q: What’s often considered the biggest benefit of using AI in manufacturing?
A: Many people point to improved operational efficiency as the most significant upside. This typically leads directly to cost savings and a boost in overall productivity.
Q: Can smaller manufacturers also benefit from AI?
A: Absolutely, yes. Small manufacturers don’t necessarily need to implement everything at once. They can often see real benefits by focusing on just one or two specific applications, like predictive maintenance for key machines or AI quality control on their main product line, to improve efficiency and reduce costs in targeted ways.
Q: Will AI replace human workers in factories?
A: This is a common question, and the general view is that AI is more likely to work alongside human employees rather than completely replace everyone. It’s great at automating repetitive, dull, or dangerous tasks and providing valuable insights that help humans make smarter decisions. The focus is often on augmenting human capabilities.
Q: What kind of data is needed for AI in manufacturing?
A: You typically need a variety of data, including sensor data from machines, historical production records, process parameters, images, and videos. The key is that this data needs to be relatively clean, accessible, and well-organized for the AI models to learn effectively.
Q: How secure is AI in manufacturing environments?
A: The security of AI in manufacturing really depends heavily on the security measures that are put in place. Implementing robust safeguards to protect both the data being used and the AI models themselves is crucial to prevent cyber threats and unauthorized access to sensitive manufacturing information.